The AI Compute Engine
Ray is at the center of the world's most powerful AI platforms. It precisely orchestrates infrastructure for any distributed workload on any accelerator at any scale.
Join Ray SlackWhy Ray?
The AI Challenge
AI grows more complex by the hour, with complex data modalities and new models, frameworks, and accelerators released daily. Without effective infrastructure, teams face slow time to production, underutilized resources, and exploding costs. To deliver real ROI and combat the AI Complexity Wall, teams need an engine to support any workload – across AI, ML, and Gen AI.
Introducing
The AI Compute Engine
To solve the AI Complexity Wall, you need an AI Compute Engine: Ray. Ray can:
The Compute Engine for Any AI Workload
Ray is Python-native, built by developers for developers.
Parallel Python Code
Ray is Python-native. Scale and distribute any Python code for use cases like simulation, backtesting, and more.
Multi-Modal Data Processing
Process structured and unstructured data including images, videos, audio, and more.
Model Training
Run distributed training, including Gen AI foundation models, time series models, and traditional AI / ML models like XGBoost at scale with 1 line of code. And yes – it’s compatible with your framework of choice.
Model Serving
Deploy models and business logic - not instances. Ray Serve offers independent scaling and fractional resources to let you get the most of the models you deploy. Get support for any ML model – from LLMs to stable diffusion models to object detection models and beyond.
Batch Inference
Leverage heterogeneous compute to streamline offline batch inference workflows. Use CPUs and GPUs in the same pipeline to increase utilization, fully saturate GPUs, and decrease costs.
Reinforcement Learning
Run best-in-class reinforcement learning workflows. Ray RLlib supports production-level, highly distributed RL workloads while maintaining unified and simple APIs for a large variety of industry applications.
Gen AI
Build end-to-end GenAI workflows with Ray. Ray supports multimodal models, RAG applications, and more.
LLM Inference
Serve Large Language Models and scale seamlessly with Ray. Ray's flexility to support any accelerator, any model, coupled with seamless scaling is built for LLM inference (online and batch).
LLM Fine Tuning
From the framework behind ChatGPT, easily fine tune Large Language models at scale.
Key Concepts in Ray
Ray is the AI Compute Engine designed to power your AI platform and optimize any workload at any scale.
Core
Scale Python Code
Ray Core provides a small number of core primitives (i.e., tasks, actors, objects) for building and scaling distributed Python applications.
Ray Libraries
End-to-End AI Solutions
Seamlessly scale any AI workload - including data processing, training, and serving - with Ray's high-level ML libraries for developers.
Ecosystem & Tools
A Full ML Ecosystem
Run end-to-end AI and ML workflows on Ray. Get easy-to-use tools to deploy Ray clusters, debug and optimize applications, and integrate with common tools and frameworks to build AI applications.
Anyscale is Ray - Perfected
Try Anyscale Today.
Real Results at (Any) Scale
See how industry leaders leverage Ray to accelerate their AI operations and unlock transformative results.
Open Source and Future Proof
Backed by a growing open source community, Ray powers today's AI leaders while evolving for tomorrow's AI innovations.
40k+
GitHub repo downloads
34.8k
stars by the community
1000+
Contributors
“Anything you can describe with functions and classes—which is pretty much every application that you write—you can distribute with Ray."
Patrick AmesPrincipal Engineer
“From my perspective [Ray] is purely magic."
Thierry SteenberghsPrincipal Software Engineer

Get Expert Help
Get hands-on training and expert support from the team that created Ray.
Anyscale is a fully managed AI platform for Ray, built by the minds behind it. Get all the benefits of Ray and many more, including enterprise governance, advanced developer tooling, and expert support. Contact us to learn more about working with the Anyscale team to develop, debug, and optimize your Ray use cases
Sign Up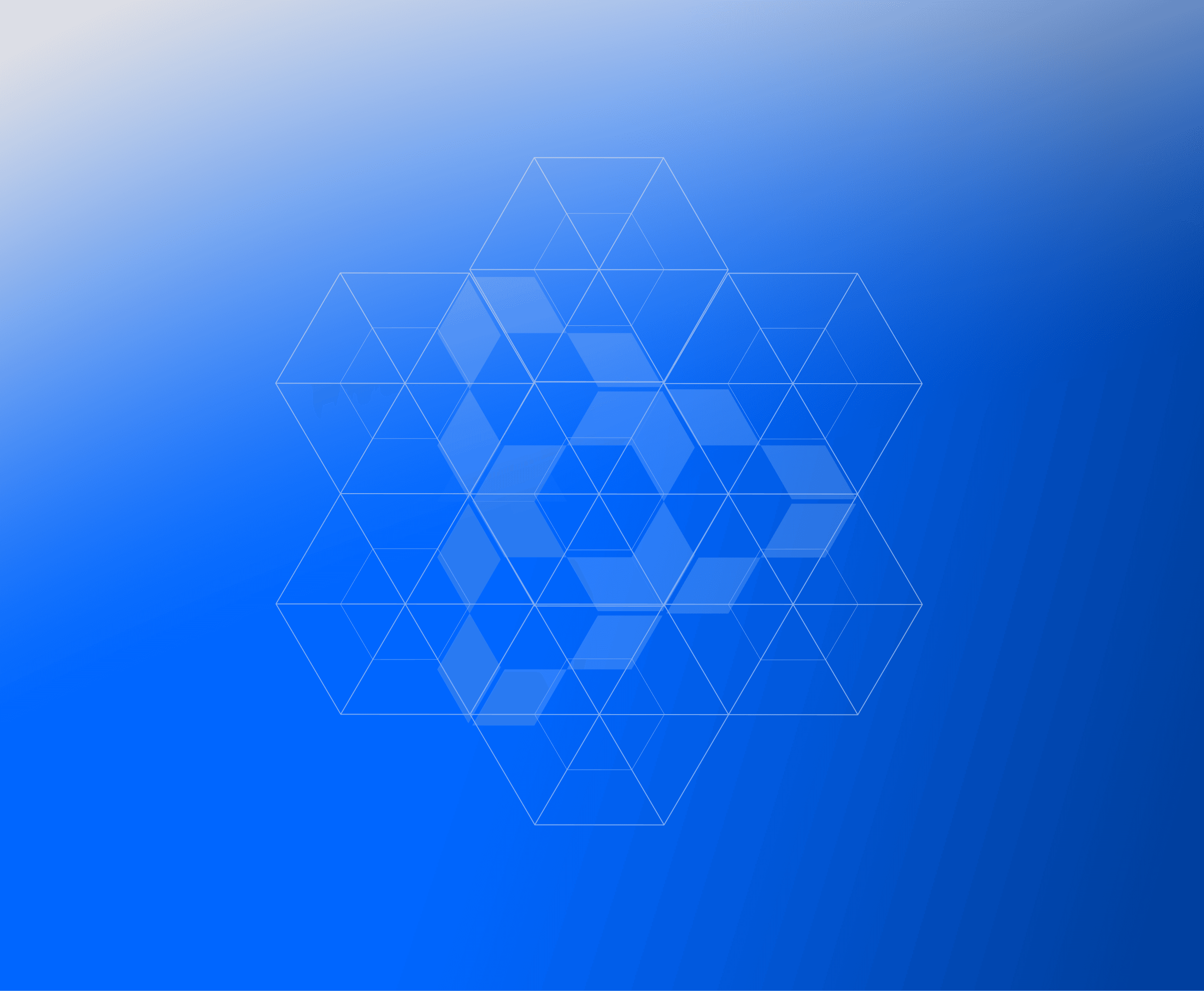
Get Started Today
Anyscale is the best place to run Ray. Accelerate your Ray journey and transform your team to an AI team with Ray and Anyscale.
Advance your AI Platform with Anyscale
Ready to truly invest in AI? See why Anyscale is chosen by the world's smartest AI/Ml platform leaders.
Ray Slack Community
Join the conversation and connect with fellow AI practitioners on the Ray Community Slack.
Try Ray on Anyscale
Get started with the only fully-managed, all-in-one platform to run Ray - built by the minds behind it.